Enhancing Organizational Security with Cloud Identity and Access Management: Exploring GCP and Okta Solutions
The future of data: Choosing a data platform and best practices of data management
According to recent Google Cloud data research, in the future, data will be unified, flexible, and easily accessible.
Data is critical to driving innovative product decisions and user experiences along with broad go-to-market strategies. Harnessing your data successfully can give you a significant competitive advantage. That’s why most tech companies and startups are investing in data management — to modernize and operate at larger and larger scales, to justify current and future data costs, and to elevate their organizational maturity and decision-making.
According to Google Cloud research, there are 3 key data approaches that innovative tech companies stick to:
- Data must be unified across the entire company, and even across suppliers and partners.
- The technology stack must be flexible enough to support use cases ranging from offline data analysis to real-time machine learning.
- The stack must also be easily accessible and must support different platforms, programming languages, tools, and open standards.
However, there are challenges surrounding access, storage, inconsistent tools, compliance, and security that make it hard to go below the surface and unlock real value from your data. Among them:
- Inherited legacy ecosystems with different technological stacks
- The decision to store your data in a single cloud or multiple clouds
- Batching or micro-batching your data today instead of processing it in real time
- Lacking easy access to all your data and missing out on the ability to process and analyze it a
We recommend two main principles for choosing a data platform that will help you solve data challenges and bring your data management to the next level.
Principle 1: Simplicity and scalability
Smaller systems have generally been simpler. However, you no longer have to choose between a system that’s easy to use and a system that’s highly scalable. Using a serverless architecture eliminates the need for cluster management and gives you the ability to handle massive scale for both compute and storage, so you never have to worry about data size exceeding your technical capacity again. For both simplicity and scalability, we recommend a serverless data platform. We suggest you discard any option that requires you to install software, manage clusters, or tune queries.
Principle 2: Agility and keeping costs down
Any data management system that combines compute and storage will force you to scale up compute to deal with increasing data volume, even if you don’t need it. This can be expensive, and you might find yourself making compromises such as only storing the last twelve months’ worth of data in your analytics warehouse.
To reduce infrastructure management as much as possible, consider a serverless, multicloud data warehouse with enhanced reliability, performance, and built-in data protection (such as BigQuery).
With something like BigQuery, you don’t need to plan queries in advance or index your data sets. Decoupled storage and compute let you land data without worrying that it’s going to drive up your querying costs, and your data scientists can experiment without having to worry about clusters or sizing their data warehouses to try new ideas through ad hoc queries.
Now after when we have reviewed the principles of choosing the right data management platform, let’s highlight some of the best practices of data management:
Make data-driven decisions in real time
You want to be able to capture data in realtime and make that data available for low-latency querying by your business teams. You also want to make sure your streaming pipelines are scalable, resilient, and have low management overhead. BigQuery has native support for ingesting streaming data and makes that data immediately available for analysis using SQL. Along with BigQuery’s easy-to-use Streaming API, Dataflow gives you the ability to manage your seasonal and spiky workloads without overspending.
Break down data silos
Many organizations end up creating silos because they store data separately across departments and business units, with each team owning its own data. This means that whenever you want to do an analysis, you have to figure out how to break down those silos. Today’s multicloud, hybrid-cloud reality requires another level of sophistication in managing and accessing siloed data.
You can land all your data in BigQuery and provide reusable functions, materialized views, and even the ability to train ML models without any data movement. This means that even non-technical domain experts (and partners and suppliers who have permission) can easily access and use SQL to query the data using familiar tools such as spreadsheets and dashboards.
Make the access to all your data easier
Historically, unstructured and semi-structured data were best served by data lakes, while structured data fit best in data warehouses. This separation created technological silos that made crossing the format divide difficult; you’d store all your data in a data lake because it’s cheaper and easier to manage, then move the data to a warehouse so you could use analytics tools to extract insights.
Are you leveraging the optimal data storage strategy for your analytics? Read our article about data lake vs data warehouse with our enlightening comparative analysis.
Use AI/ML to experiment faster and manage workloads
If you’re serious about differentiating based on data, you want to extract the highest value you can from the data you’re collecting. To do that, you want your data science teams to be as productive as possible and not miss opportunities.
The quality of your pre-built and low-code models is crucial. AutoML on Vertex AI makes best-of-class AI models available in a no-code environment, which allows for fast benchmarking and prioritization.
To drive real value in production, systems must be able to ingest, process, and serve data, and machine learning must drive personalized services in real time based on the customer’s context.
As data-driven decision making becomes the norm, platforms like Looker become essential tools for businesses. Looker offers advanced features for data exploration, analysis, and visualization. However, ensuring your data platform choice (see Looker vs Tableau) and Looker implementation are future-proof requires expertise. A Looker consultant can provide the guidance you need to navigate the ever-evolving data landscape and leverage Looker’s capabilities effectively.
We’ve talked a lot about harnessing your data and what that really means, along with some considerations you might face while migrating to a data warehouse in the cloud.
To learn more about how Google Cloud can help you use insights to gain a significant business advantage – you may contact the official Google Cloud Premier Partner – Cloudfresh.
Cloudfresh team is a unique center of expertise for Google Cloud, Zendesk, CloudM and Asana. For these products, we can provide you with the following services:
- Customization;
- Development;
- Integration;
- Training;
- License;
- Support.
Our specialists will help you optimize your IT infrastructure, develop integrations for better system interoperability, and help create completely new structures and processes for your teams, while our support center will provide you with the best customer experience!
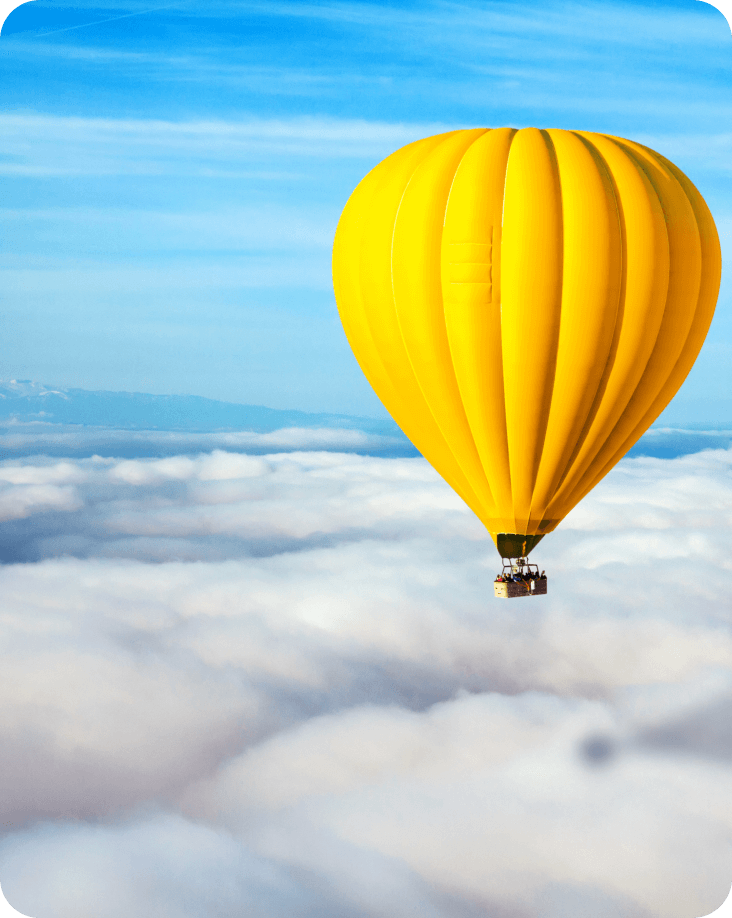